Part I: How Industries Fail
Until three years ago, the oldest company in the world was the construction company Kongo Gumi, headquartered in Osaka, Japan. Kongo Gumi was founded in 578 CE when the then-regent of Japan, Prince Shotoku, brought a member of the Kongo family from Korea to Japan to help construct the first Buddhist temple in Japan, the Shitenno-ji. The Kongo Gumi continued in the construction trade for almost one and a half thousand years. In 2005, they were headed by Masakazu Kongo, the 40th of his family to head Kongo Gumi. The company had more than 100 employees, and 70 million dollars in revenue. But in 2006, Kongo Gumi went into liquidation, and its assets were purchased by Takamatsu Corporation. Kongo Gumi as an independent entity no longer exists.
How is it that large, powerful organizations, with access to vast sums of money, and many talented, hardworking people, can simply disappear? Examples abound – consider General Motors, Lehman Brothers and MCI Worldcom – but the question is most fascinating when it is not just a single company that goes bankrupt, but rather an entire industry is disrupted. In the 1970s, for example, some of the world’s fastest-growing companies were companies like Digital Equipment Corporation, Data General and Prime. They made minicomputers like the legendary PDP-11. None of these companies exist today. A similar disruption is happening now in many media industries. CD sales peaked in 2000, shortly after Napster started, and have declined almost 30 percent since. Newspaper advertising revenue in the United States has declined 30 percent in the last 3 years, and the decline is accelerating: one third of that fall came in the last quarter.
There are two common explanations for the disruption of industries like minicomputers, music, and newspapers. The first explanation is essentially that the people in charge of the failing industries are stupid. How else could it be, the argument goes, that those enormous companies, with all that money and expertise, failed to see that services like iTunes and Last.fm are the wave of the future? Why did they not pre-empt those services by creating similar products of their own? Polite critics phrase their explanations less bluntly, but nonetheless many explanations boil down to a presumption of stupidity. The second common explanation for the failure of an entire industry is that the people in charge are malevolent. In that explanation, evil record company and newspaper executives have been screwing over their customers for years, simply to preserve a status quo that they personally find comfortable.
It’s true that stupidity and malevolence do sometimes play a role in the disruption of industries. But in the first part of this essay I’ll argue that even smart and good organizations can fail in the face of disruptive change, and that there are common underlying structural reasons why that’s the case. That’s a much scarier story. If you think the newspapers and record companies are stupid or malevolent, then you can reassure yourself that provided you’re smart and good, you don’t have anything to worry about. But if disruption can destroy even the smart and the good, then it can destroy anybody. In the second part of the essay, I’ll argue that scientific publishing is in the early days of a major disruption, with similar underlying causes, and will change radically over the next few years.
Why online news is killing the newspapers
To make our discussion of disruption concrete, let’s think about why many blogs are thriving financially, while the newspapers are dying. This subject has been discussed extensively in many recent articles, but my discussion is different because it focuses on identifying general structural features that don’t just explain the disruption of newspapers, but can also help explain other disruptions, like the collapse of the minicomputer and music industries, and the impending disruption of scientific publishing.
Some people explain the slow death of newspapers by saying that blogs and other online sources [1] are news parasites, feeding off the original reporting done by the newspapers. That’s false. While it’s true that many blogs don’t do original reporting, it’s equally true that many of the top blogs do excellent original reporting. A good example is the popular technology blog TechCrunch, by most measures one of the top 100 blogs in the world. Started by Michael Arrington in 2005, TechCrunch has rapidly grown, and now employs a large staff. Part of the reason it’s grown is because TechCrunch’s reporting is some of the best in the technology industry, comparable to, say, the technology reporting in the New York Times. Yet whereas the New York Times is wilting financially [2], TechCrunch is thriving, because TechCrunch’s operating costs are far lower, per word, than the New York Times. The result is that not only is the audience for technology news moving away from the technology section of newspapers and toward blogs like TechCrunch, the blogs can undercut the newspaper’s advertising rates. This depresses the price of advertising and causes the advertisers to move away from the newspapers.
Unfortunately for the newspapers, there’s little they can do to make themselves cheaper to run. To see why that is, let’s zoom in on just one aspect of newspapers: photography. If you’ve ever been interviewed for a story in the newspaper, chances are a photographer accompanied the reporter. You get interviewed, the photographer takes some snaps, and the photo may or may not show up in the paper. Between the money paid to the photographer and all the other costs, that photo probably costs the newspaper on the order of a few hundred dollars [3]. When TechCrunch or a similar blog needs a photo for a post, they’ll use a stock photo, or ask their subject to send them a snap, or whatever. The average cost is probably tens of dollars. Voila! An order of magnitude or more decrease in costs for the photo.
Here’s the kicker. TechCrunch isn’t being any smarter than the newspapers. It’s not as though no-one at the newspapers ever thought “Hey, why don’t we ask interviewees to send us a polaroid, and save some money?” Newspapers employ photographers for an excellent business reason: good quality photography is a distinguishing feature that can help establish a superior newspaper brand. For a high-end paper, it’s probably historically been worth millions of dollars to get stunning, Pulitzer Prizewinning photography. It makes complete business sense to spend a few hundred dollars per photo.
What can you do, as a newspaper editor? You could fire your staff photographers. But if you do that, you’ll destroy the morale not just of the photographers, but of all your staff. You’ll stir up the Unions. You’ll give a competitive advantage to your newspaper competitors. And, at the end of the day, you’ll still be paying far more per word for news than TechCrunch, and the quality of your product will be no more competitive.
The problem is that your newspaper has an organizational architecture which is, to use the physicists’ phrase, a local optimum. Relatively small changes to that architecture – like firing your photographers – don’t make your situation better, they make it worse. So you’re stuck gazing over at TechCrunch, who is at an even better local optimum, a local optimum that could not have existed twenty years ago:
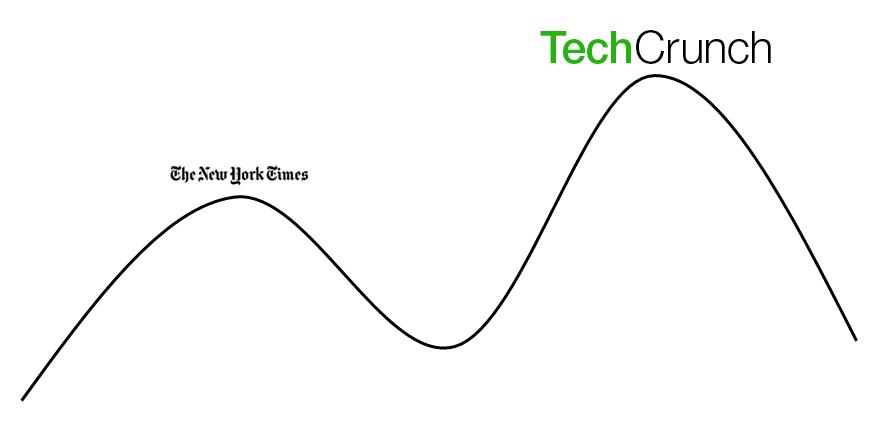
Unfortunately for you, there’s no way you can get to that new optimum without attempting passage through a deep and unfriendly valley. The incremental actions needed to get there would be hell on the newspaper. There’s a good chance they’d lead the Board to fire you.
The result is that the newspapers are locked into producing a product that’s of comparable quality (from an advertiser’s point of view) to the top blogs, but at far greater cost. And yet all their decisions – like the decision to spend a lot on photography – are entirely sensible business decisions. Even if they’re smart and good, they’re caught on the horns of a cruel dilemma.
The same basic story can be told about the dispruption of the music industry, the minicomputer industry, and many other disruptions. Each industry has (or had) a standard organizational architecture. That organizational architecture is close to optimal, in the sense that small changes mostly make things worse, not better. Everyone in the industry uses some close variant of that architecture. Then a new technology emerges and creates the possibility for a radically different organizational architecture, using an entirely different combination of skills and relationships. The only way to get from one organizational architecture to the other is to make drastic, painful changes. The money and power that come from commitment to an existing organizational architecture actually place incumbents at a disadvantage, locking them in. It’s easier and more effective to start over, from scratch.
Organizational immune systems
I’ve described why it’s hard for incumbent organizations in a disrupted industry to change to a new model. The situation is even worse than I’ve described so far, though, because some of the forces preventing change are strongest in the best run organizations. The reason is that those organizations are large, complex structures, and to survive and prosper they must contain a sort of organizational immune system dedicated to preserving that structure. If they didn’t have such an immune system, they’d fall apart in the ordinary course of events. Most of the time the immune system is a good thing, a way of preserving what’s good about an organization, and at the same time allowing healthy gradual change. But when an organization needs catastrophic gut-wrenching change to stay alive, the immune system becomes a liability.
To see how such an immune system expresses itself, imagine someone at the New York Times had tried to start a service like Google News, prior to Google News. Even before the product launched they would have been constantly attacked from within the organization for promoting competitors’ products. They would likely have been forced to water down and distort the service, probably to the point where it was nearly useless for potential customers. And even if they’d managed to win the internal fight and launched a product that wasn’t watered down, they would then have been attacked viciously by the New York Times’ competitors, who would suspect a ploy to steal business. Only someone outside the industry could have launched a service like Google News.
Another example of the immune response is all the recent news pieces lamenting the death of newspapers. Here’s one such piece, from the Editor of the New York Times’ editorial page, Andrew Rosenthal:
There’s a great deal of good commentary out there on the Web, as you say. Frankly, I think it is the task of bloggers to catch up to us, not the other way around… Our board is staffed with people with a wide and deep range of knowledge on many subjects. Phil Boffey, for example, has decades of science and medical writing under his belt and often writes on those issues for us… Here’s one way to look at it: If the Times editorial board were a single person, he or she would have six Pulitzer prizes…
This is a classic immune response. It demonstrates a deep commitment to high-quality journalism, and the other values that have made the New York Times great. In ordinary times this kind of commitment to values would be a sign of strength. The problem is that as good as Phil Boffey might be, I prefer the combined talents of Fields medallist Terry Tao, Nobel prize winner Carl Wieman, MacArthur Fellow Luis von Ahn, acclaimed science writer Carl Zimmer, and thousands of others. The blogosophere has at least four Fields medallists (the Nobel of math), three Nobelists, and many more luminaries. The New York Times can keep its Pulitzer Prizes. Other lamentations about the death of newspapers show similar signs of being an immune response. These people aren’t stupid or malevolent. They’re the best people in the business, people who are smart, good at their jobs, and well-intentioned. They are, in short, the people who have most strongly internalized the values, norms and collective knowledge of their industry, and thus have the strongest immune response. That’s why the last people to know an industry is dead are the people in it. I wonder if Andrew Rosenthal and his colleagues understand that someone equipped with an RSS reader can assemble a set of news feeds that renders the New York Times virtually irrelevant? If a person inside an industry needs to frequently explain why it’s not dead, they’re almost certainly wrong.
What are the signs of impending disruption?
Five years ago, most newspaper editors would have laughed at the idea that blogs might one day offer serious competition. The minicomputer companies laughed at the early personal computers. New technologies often don’t look very good in their early stages, and that means a straightup comparison of new to old is little help in recognizing impending dispruption. That’s a problem, though, because the best time to recognize disruption is in its early stages. The journalists and newspaper editors who’ve only recognized their problems in the last three to four years are sunk. They needed to recognize the impending disruption back before blogs looked like serious competitors, when evaluated in conventional terms.
An early sign of impending disruption is when there’s a sudden flourishing of startup organizations serving an overlapping customer need (say, news), but whose organizational architecture is radically different to the conventional approach. That means many people outside the old industry (and thus not suffering from the blinders of an immune response) are willing to bet large sums of their own money on a new way of doing things. That’s exactly what we saw in the period 2000-2005, with organizations like Slashdot, Digg, Fark, Reddit, Talking Points Memo, and many others. Most such startups die. That’s okay: it’s how the new industry learns what organizational architectures work, and what don’t. But if even a few of the startups do okay, then the old players are in trouble, because the startups have far more room for improvement.
Part II: Is scientific publishing about to be disrupted?
What’s all this got to do with scientific publishing? Today, scientific publishers are production companies, specializing in services like editorial, copyediting, and, in some cases, sales and marketing. My claim is that in ten to twenty years, scientific publishers will be technology companies [4]. By this, I don’t just mean that they’ll be heavy users of technology, or employ a large IT staff. I mean they’ll be technology-driven companies in a similar way to, say, Google or Apple. That is, their foundation will be technological innovation, and most key decision-makers will be people with deep technological expertise. Those publishers that don’t become technology driven will die off.
Predictions that scientific publishing is about to be disrupted are not new. In the late 1990s, many people speculated that the publishers might be in trouble, as free online preprint servers became increasingly popular in parts of science like physics. Surely, the argument went, the widespread use of preprints meant that the need for journals would diminish. But so far, that hasn’t happened. Why it hasn’t happened is a fascinating story, which I’ve discussed in part elsewhere, and I won’t repeat that discussion here.
What I will do instead is draw your attention to a striking difference between today’s scientific publishing landscape, and the landscape of ten years ago. What’s new today is the flourishing of an ecosystem of startups that are experimenting with new ways of communicating research, some radically different to conventional journals. Consider Chemspider, the excellent online database of more than 20 million molecules, recently acquired by the Royal Society of Chemistry. Consider Mendeley, a platform for managing, filtering and searching scientific papers, with backing from some of the people involved in Last.fm and Skype. Or consider startups like SciVee (YouTube for scientists), the Public Library of Science, the Journal of Visualized Experiments, vibrant community sites like OpenWetWare and the Alzheimer Research Forum, and dozens more. And then there are companies like WordPress, Friendfeed, and Wikimedia, that weren’t started with science in mind, but which are increasingly helping scientists communicate their research. This flourishing ecosystem is not too dissimilar from the sudden flourishing of online news services we saw over the period 2000 to 2005.
Let’s look up close at one element of this flourishing ecosystem: the gradual rise of science blogs as a serious medium for research. It’s easy to miss the impact of blogs on research, because most science blogs focus on outreach. But more and more blogs contain high quality research content. Look at Terry Tao’s wonderful series of posts explaining one of the biggest breakthroughs in recent mathematical history, the proof of the Poincare conjecture. Or Tim Gowers recent experiment in “massively collaborative mathematics”, using open source principles to successfully attack a significant mathematical problem. Or Richard Lipton’s excellent series of posts exploring his ideas for solving a major problem in computer science, namely, finding a fast algorithm for factoring large numbers. Scientific publishers should be terrified that some of the world’s best scientists, people at or near their research peak, people whose time is at a premium, are spending hundreds of hours each year creating original research content for their blogs, content that in many cases would be difficult or impossible to publish in a conventional journal. What we’re seeing here is a spectacular expansion in the range of the blog medium. By comparison, the journals are standing still.
This flourishing ecosystem of startups is just one sign that scientific publishing is moving from being a production industry to a technology industry. A second sign of this move is that the nature of information is changing. Until the late 20th century, information was a static entity. The natural way for publishers in all media to add value was through production and distribution, and so they employed people skilled in those tasks, and in supporting tasks like sales and marketing. But the cost of distributing information has now dropped almost to zero, and production and content costs have also dropped radically [5]. At the same time, the world’s information is now rapidly being put into a single, active network, where it can wake up and come alive. The result is that the people who add the most value to information are no longer the people who do production and distribution. Instead, it’s the technology people, the programmers.
If you doubt this, look at where the profits are migrating in other media industries. In music, they’re migrating to organizations like Apple. In books, they’re migrating to organizations like Amazon, with the Kindle. In many other areas of media, they’re migrating to Google: Google is becoming the world’s largest media company. They don’t describe themselves that way (see also here), but the media industry’s profits are certainly moving to Google. All these organizations are run by people with deep technical expertise. How many scientific publishers are run by people who know the difference between an INNER JOIN and an OUTER JOIN? Or who know what an A/B test is? Or who know how to set up a Hadoop cluster? Without technical knowledge of this type it’s impossible to run a technology-driven organization. How many scientific publishers are as knowledgeable about technology as Steve Jobs, Sergey Brin, or Larry Page?
I expect few scientific publishers will believe and act on predictions of disruption. One common response to such predictions is the appealing game of comparison: “but we’re better than blogs / wikis / PLoS One / …!” These statements are currently true, at least when judged according to the conventional values of scientific publishing. But they’re as irrelevant as the equally true analogous statements were for newspapers. It’s also easy to vent standard immune responses: “but what about peer review”, “what about quality control”, “how will scientists know what to read”. These questions express important values, but to get hung up on them suggests a lack of imagination much like Andrew Rosenthal’s defense of the New York Times editorial page. (I sometimes wonder how many journal editors still use Yahoo!’s human curated topic directory instead of Google?) In conversations with editors I repeatedly encounter the same pattern: “But idea X won’t work / shouldn’t be allowed / is bad because of Y.” Well, okay. So what? If you’re right, you’ll be intellectually vindicated, and can take a bow. If you’re wrong, your company may not exist in ten years. Whether you’re right or not is not the point. When new technologies are being developed, the organizations that win are those that aggressively take risks, put visionary technologists in key decision-making positions, attain a deep organizational mastery of the relevant technologies, and, in most cases, make a lot of mistakes. Being wrong is a feature, not a bug, if it helps you evolve a model that works: you start out with an idea that’s just plain wrong, but that contains the seed of a better idea. You improve it, and you’re only somewhat wrong. You improve it again, and you end up the only game in town. Unfortunately, few scientific publishers are attempting to become technology-driven in this way. The only major examples I know of are Nature Publishing Group (with Nature.com) and the Public Library of Science. Many other publishers are experimenting with technology, but those experiments remain under the control of people whose core expertise is in others areas.
Opportunities
So far this essay has focused on the existing scientific publishers, and it’s been rather pessimistic. But of course that pessimism is just a tiny part of an exciting story about the opportunities we have to develop new ways of structuring and communicating scientific information. These opportunities can still be grasped by scientific publishers who are willing to let go and become technology-driven, even when that threatens to extinguish their old way of doing things. And, as we’ve seen, these opportunites are and will be grasped by bold entrepreneurs. Here’s a list of services I expect to see developed over the next few years. A few of these ideas are already under development, mostly by startups, but have yet to reach the quality level needed to become ubiquitous. The list could easily be continued ad nauseum – these are just a few of the more obvious things to do.
Personalized paper recommendations: Amazon.com has had this for books since the late 1990s. You go to the site and rate your favourite books. The system identifies people with similar taste, and automatically constructs a list of recommendations for you. This is not difficult to do: Amazon has published an early variant of its algorithm, and there’s an entire ecosystem of work, much of it public, stimulated by the Neflix Prize for movie recommendations. If you look in the original Google PageRank paper, you’ll discover that the paper describes a personalized version of PageRank, which can be used to build a personalized search and recommendation system. Google doesn’t actually use the personalized algorithm, because it’s far more computationally intensive than ordinary PageRank, and even for Google it’s hard to scale to tens of billions of webpages. But if all you’re trying to rank is (say) the physics literature – a few million papers – then it turns out that with a little ingenuity you can implement personalized PageRank on a small cluster of computers. It’s possible this can be used to build a system even better than Amazon or Netflix.
A great search engine for science: ISI’s Web of Knowledge, Elsevier’s Scopus and Google Scholar are remarkable tools, but there’s still huge scope to extend and improve scientific search engines [6]. With a few exceptions, they don’t do even basic things like automatic spelling correction, good relevancy ranking of papers (preferably personalized), automated translation, or decent alerting services. They certainly don’t do more advanced things, like providing social features, or strong automated tools for data mining. Why not have a public API [7] so people can build their own applications to extract value out of the scientific literature? Imagine using techniques from machine learning to automatically identify underappreciated papers, or to identify emerging areas of study.
High-quality tools for real-time collaboration by scientists: Look at services like the collaborative editor Etherpad, which lets multiple people edit a document, in real time, through the browser. They’re even developing a feature allowing you to play back the editing process. Or the similar service from Google, Google Docs, which also offers shared spreadsheets and presentations. Look at social version control systems like Git and Github. Or visualization tools which let you track different people’s contributions. These are just a few of hundreds of general purpose collaborative tools that are lightyears beyond what scientists use. They’re not widely adopted by scientists yet, in part for superficial reasons: they don’t integrate with things like LaTeX and standard bibliographical tools. Yet achieving that kind of integration is trivial compared with the problems these tools do solve. Looking beyond, services like Google Wave may be a platform for startups to build a suite of collaboration clients that every scientist in the world will eventually use.
Scientific blogging and wiki platforms: With the exception of Nature Publishing Group, why aren’t the scientific publishers developing high-quality scientific blogging and wiki platforms? It would be easy to build upon the open source WordPress platform, for example, setting up a hosting service that makes it easy for scientists to set up a blog, and adds important features not present in a standard WordPress installation, like reliable signing of posts, timestamping, human-readable URLs, and support for multiple post versions, with the ability to see (and cite) a full revision history. A commenter-identity system could be created that enabled filtering and aggregation of comments. Perhaps most importantly, blog posts could be made fully citable.
On a related note, publishers could also help preserve some of the important work now being done on scientific blogs and wikis. Projects like Tim Gowers’ Polymath Project are an important part of the scientific record, but where is the record of work going to be stored in 10 or 20 years time? The US Library of Congress has taken the initiative in preserving law blogs. Someone needs to step up and do the same for science blogs.
The data web: Where are the services making it as simple and easy for scientists to publish data as it to publish a journal paper or start a blog? A few scientific publishers are taking steps in this direction. But it’s not enough to just dump data on the web. It needs to be organized and searchable, so people can find and use it. The data needs to be linked, as the utility of data sets grows in proportion to the connections between them. It needs to be citable. And there needs to be simple, easy-to-use infrastructure and expertise to extract value from that data. On every single one of these issues, publishers are at risk of being leapfrogged by companies like Metaweb, who are building platforms for the data web.
Why many services will fail: Many unsuccessful attempts at implementing services like those I’ve just described have been made. I’ve had journal editors explain to me that this shows there is no need for such services. I think in many cases there’s a much simpler explanation: poor execution [8]. Development projects are often led by senior editors or senior scientists whose hands-on technical knowledge is minimal, and whose day-to-day involvement is sporadic. Implementation is instead delegated to IT-underlings with little power. It should surprise no one that the results are often mediocre. Developing high-quality web services requires deep knowledge and drive. The people who succeed at doing it are usually brilliant and deeply technically knowledgeable. Yet it’s surprisingly common to find projects being led by senior scientists or senior editors whose main claim to “expertise” is that they wrote a few programs while a grad student or postdoc, and who now think they can get a high-quality result with minimal extra technical knowledge. That’s not what it means to be technology-driven.
Conclusion: I’ve presented a pessimistic view of the future of current scientific publishers. Yet I hope it’s also clear that there are enormous opportunities to innovate, for those willing to master new technologies, and to experiment boldly with new ways of doing things. The result will be a great wave of innovation that changes not just how scientific discoveries are communicated, but also accelerates the way scientific discoveries are made.
Notes
[1] We’ll focus on blogs to make the discussion concrete, but in fact many new forms of media are contributing to the newspapers’ decline, including news sites like Digg and MetaFilter, analysis sites like Stratfor, and many others. When I write “blogs” in what follows I’m usually referring to this larger class of disruptive new media, not literally to conventional blogs, per se.
[2] In a way, it’s ironic that I use the New York Times as an example. Although the New York Times is certainly going to have a lot of trouble over the next five years, in the long run I think they are one of the newspapers most likely to survive: they produce high-quality original content, show strong signs of becoming technology driven, and are experimenting boldly with alternate sources of content. But they need to survive the great newspaper die-off that’s coming over the next five or so years.
[3] In an earlier version of this essay I used the figure 1,000 dollars. That was sloppy – it’s certainly too high. The actual figure will certainly vary quite a lot from paper to paper, but for a major newspaper in a big city I think on the order of 200-300 dollars is a reasonable estimate, when all costs are factored in.
[4] I’ll use the term “companies” to include for-profit and not-for-profit organizations, as well as other organizational forms. Note that the physics preprint arXiv is arguably the most successful publisher in physics, yet is neither a conventional for-profit or not-for-profit organization.
[5] This drop in production and distribution costs is directly related to the current move toward open access publication of scientific papers. This movement is one of the first visible symptoms of the disruption of scientific publishing. Much more can and has been said about the impact of open access on publishing; rather than review that material, I refer you to the blog “Open Access News”, and in particular to Peter Suber’s overview of open access.
[6] In the first version of this essay I wrote that the existing services were “mediocre”. That’s wrong, and unfair: they’re very useful services. But there’s a lot of scope for improvement.
[7] After posting this essay, Christina Pikas pointed out that Web of Science and Scopus do have APIs. That’s my mistake, and something I didn’t know.
[8] There are also services where the primary problem is cultural barriers. But for the ideas I’ve described cultural barriers are only a small part of the problem.
Acknowledgments: Thanks to Jen Dodd and Ilya Grigorik for many enlightening discussions.
About this essay: This essay is based on a colloquium given June 11, 2009, at the American Physical Society Editorial Offices. Many thanks to the people at the APS for being great hosts, and for many stimulating conversations.
Further reading:
Some of the ideas explored in this essay are developed at greater length in my book Reinventing Discovery: The New Era of Networked Science.
You can subscribe to my blog here.
My account of how industries fail was influenced by and complements Clayton Christensen’s book “The Innovator’s Dilemma”. Three of my favourite blogs about the future of scientific communication are “Science in the Open”, “Open Access News” and “Common Knowledge”. Of course, there are many more excellent sources of information on this topic. A good source aggregating these many sources is the Science 2.0 room on FriendFeed.